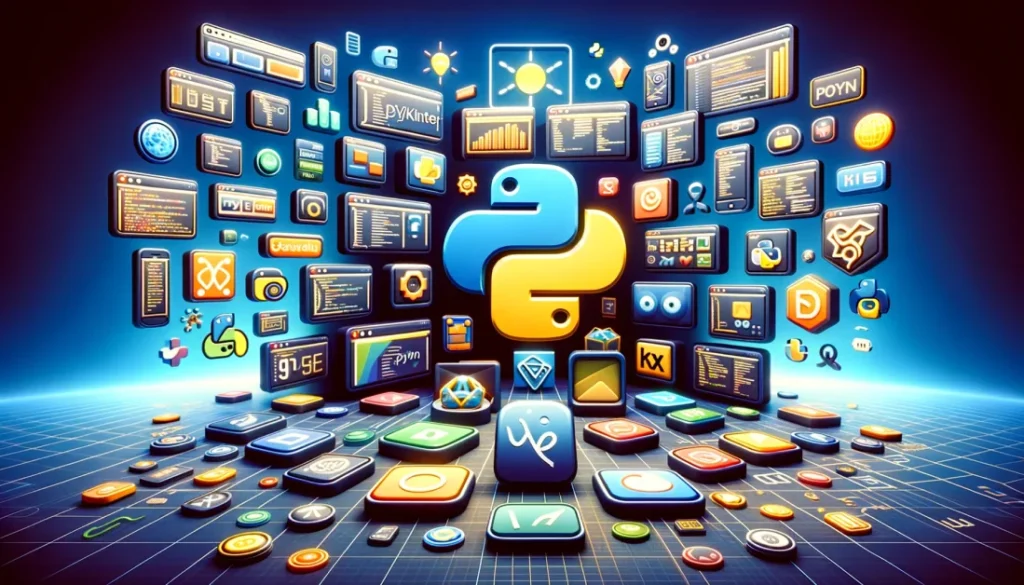
Python for AI is pretty much a classic choice – much like PB & jelly for bread or shoes to match a bag. It’s also why Python is a great pick to start the AI developer path with: simple and versatile, it still opens up new avenues for innovation and career growth for young and mid developers. In this article, we’ll review Python’s benefits more thoroughly as an AI-oriented language, trying to give you more useful tips on the way.
Why Python is the Language of Choice for AI
There’s a reason Python is that classic: it’s primarily thanks to its ease of use, readability, and extensive support libraries. Let’s elaborate on each quality.
- Simplicity and Readability: Python’s straightforward syntax allows developers to write and understand code quickly, making it accessible for AI beginners.
- Extensive Libraries and Frameworks: Python boasts a rich ecosystem of libraries and frameworks such as TensorFlow, Keras, PyTorch, and Sci-kit-learn that streamline AI and machine learning (ML) development.
- Community Support: Python has a robust and active community that contributes to a wealth of resources, tutorials, and forums, making problem-solving more straightforward for developers.
- Integration Capabilities: Python easily integrates with other languages and tools, enhancing its utility in diverse AI applications.
Getting Started with Python for AI: Essential Libraries and Tools
Just like there’s an alphabet in any language, there’s a set of must-have libraries and frameworks in programming languages. In Python, this list constitutes the following:
- NumPy: A fundamental package for scientific computing with Python. It supports large, multi-dimensional arrays and matrices, along with a collection of mathematical functions.
- Pandas: A data manipulation and analysis library that provides data structures and functions needed to work with structured data seamlessly.
- Matplotlib: A plotting library that helps visualize data through comprehensive charts and graphs.
- Sci-kit-learn: A machine learning library that offers simple and efficient tools for data mining and data analysis.
- TensorFlow and Keras: Open-source libraries developed by Google that facilitate deep learning and neural network construction.
- PyTorch: An open-source machine learning library developed by Facebook’s AI Research lab, known for its flexibility and ease of use.
Basic AI Concepts Explained Using Python Examples
Understanding basic AI concepts is crucial for any ambitious AI developer. Here are some fundamental concepts explained with Python:
1. Linear Regression
Linear regression is a method to predict a target variable based on input variables. Here’s a simple example using Sci-kit-learn:
```python
import numpy as np
import pandas as pd
from sklearn.linear_model import LinearRegression
Sample Data
data = {
'Hours Studied': [1, 2, 3, 4, 5],
'Scores': [10, 20, 30, 40, 50]
}
DataFrame
df = pd.DataFrame(data)
Variables
X = df[['Hours Studied']]
y = df['Scores']
Model
model = LinearRegression()
model.fit(X, y)
Prediction
pred = model.predict([[6]])
print(f'Predicted Score for 6 hours of study: {pred[0]}')
```
2. Classification
Classification tasks involve predicting a class label. Here’s an example using the Iris dataset with Sci-kit-learn:
```python
from sklearn.datasets import load_iris
from sklearn.model_selection import train_test_split
from sklearn.neighbors import KNeighborsClassifier
Load Dataset
iris = load_iris()
X = iris.data
y = iris.target
Split Data
X_train, X_test, y_train, y_test = train_test_split(X, y, test_size=0.3)
Model
knn = KNeighborsClassifier(n_neighbors=3)
knn.fit(X_train, y_train)
Prediction
pred = knn.predict(X_test)
print(f'Predictions: {pred}')
```
Advanced AI Topics and Python’s Role in Facilitating Complex AI Development
As you become comfortable with basic AI concepts, you’ll gradually unlock more advanced topics such as deep learning, natural language processing (NLP), and reinforcement learning.
Deep Learning
Deep learning involves neural networks with three or more layers. TensorFlow and Keras are popular libraries for building deep learning models. Here is a basic neural network example using Keras:
```python
from keras.models import Sequential
from keras.layers import Dense
Model
model = Sequential()
model.add(Dense(12, input_dim=8, activation='relu'))
model.add(Dense(8, activation='relu'))
model.add(Dense(1, activation='sigmoid'))
Compile
model.compile(loss='binary_crossentropy', optimizer='adam', metrics=['accuracy'])
Fit
model.fit(X_train, y_train, epochs=150, batch_size=10)
```
Real-world AI Projects Built with Python
Python’s role in real-world AI projects is expansive, from self-driving cars to intelligent personal assistants. Here are a few notable examples:
- Tesla’s Autopilot: Tesla uses Python extensively for developing and testing its autonomous driving software. The language aids in processing large amounts of driving data, enabling the creation of sophisticated algorithms that allow vehicles to navigate and make decisions without human intervention.
- Spotify’s Music Recommendation System: Spotify leverages Python to analyze vast amounts of user data, including listening habits and preferences. This data analysis enables Spotify to recommend personalized music playlists, enhancing the user experience by delivering music that aligns with individual tastes.
- Google’s Search Algorithms: Google employs Python to improve its search algorithms, ensuring users receive the most relevant and accurate search results. Python’s versatility and efficiency help Google process and analyze enormous data sets, refining the algorithms that power its search engine and deliver timely information to users.
The Future of AI and Python’s Role in Shaping It
Python will likely hold the fort of AI development as more groundbreaking involvements emerge; after all, it’s the combination of its qualities like simplicity, versatility, and robust ecosystem that keep this language at the top position. While the nature of AI-based inventions might shift, the leading programming language is unlikely to.
Conclusion and Next Steps
First things first: to build a strong foundation of AI development with Python, one has to understand its basics and leverage essential libraries (NumPy, Pandas, and TensorFlow). Having gained this knowledge, experiment with real-world projects: start with taking theoretical knowledge and applying it to practical scenarios. This process helps in gaining hands-on experience, understanding the complexities of real-world applications, and developing problem-solving skills that are crucial for professional growth. To further your skills, consider enrolling in an introduction to AI with Python free course and joining relevant communities where you can share knowledge and collaborate with like-minded individuals.
Ready to dive deeper into AI with Python? Start exploring today and unlock the endless possibilities that this powerful combination offers. Whether you’re aiming to enhance your career or innovate in your field, mastering AI with Python is a step toward a promising future.
Devox Software is more than just another Python web development company — it’s a team of experts specializing in the development of innovative AI solutions. With in-depth experience in Python frameworks such as TensorFlow, PyTorch and Matplotlib, they develop customized applications that meet the highest industry standards. Whether it’s developing machine learning models or delivering scalable software, Devox excels at combining technical precision and innovative thinking to meet the unique needs of each client.